Data Modernization
Data modernization is one of the most difficult to establish elements of any digital transformation.
Today, your business seeks to unlock value from its’ data in real-time and monetize it as well, but current data systems were purposely built to only store (or warehouse) information using technology that is 30 years old.
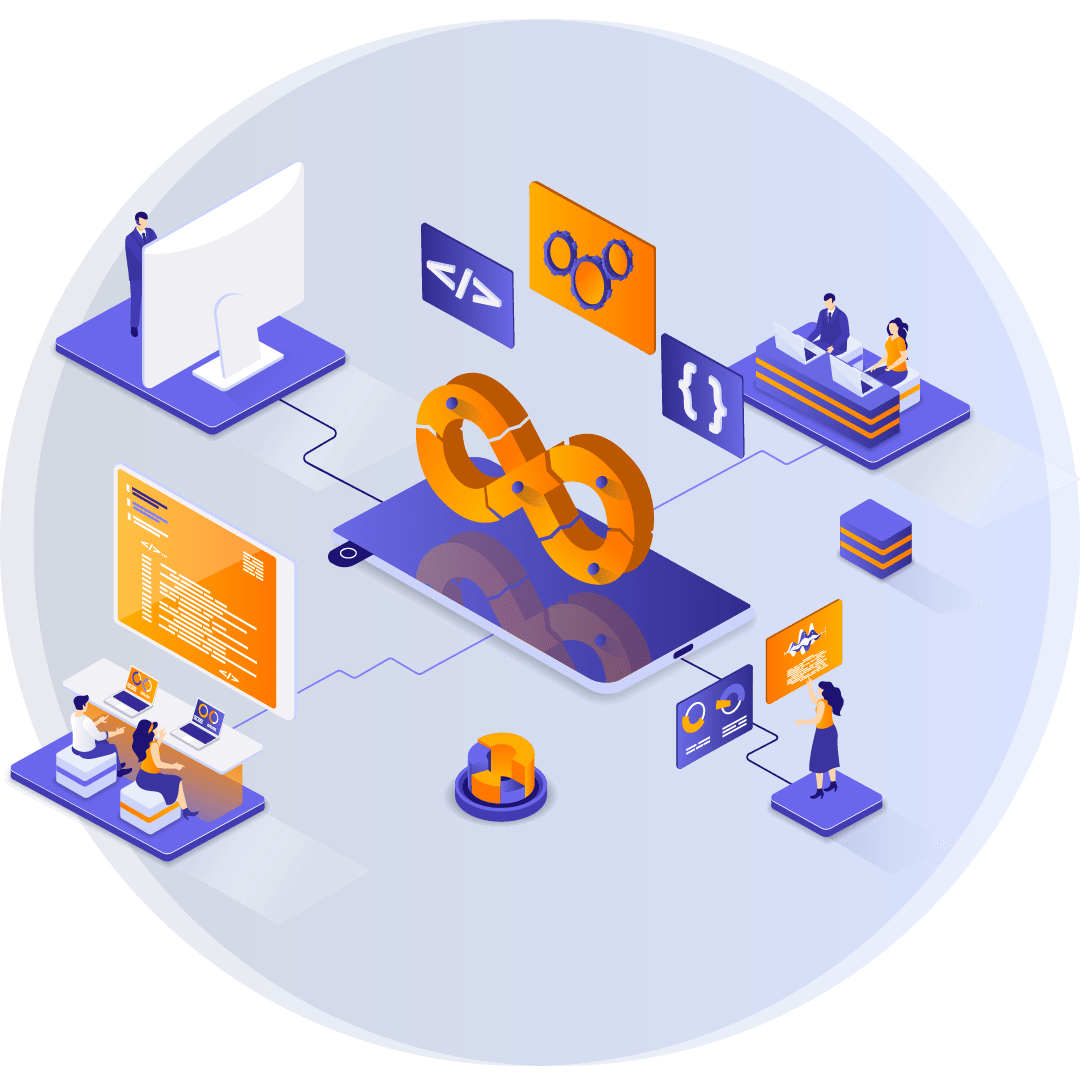
Sounds familiar?
A robust foundation of data extraction, integration, and governance is required to achieve modernization - whether it is a full-scale migration or intelligently automating processes with machine learning. Data engineering entails numerous tasks, such as building and maintaining tools, infrastructure, frameworks, and data management.
Blueshift’s Modern Data Platform enables businesses to develop advanced solutions that drive businesses forward. Our approach to data engineering includes modern data architecture, emerging technologies, and innovation across your organization, enabling you to be a digital leader within your industry.
Business Challenges
Companies commonly face challenges when it comes to executing data engineering and implementing innovative digital technologies into their business processes.
User Experience
Systems are difficult to consume and access data

Time-consuming data access
Without organised and efficient data management, enterprises spend enormous time and resources in order to access and consume their data; with the results that do not meet expectations.

Lack of self-service automation
Self-service allows users focus their time and energy on making decisions using data, rather than manual intervening through repetitive report generation tasks conducted by business users.

Incomplete story telling
User need to create a composite view of the data across multiple heterogeneous systems and services to tell a complete story - otherwise users assemble a view using Excel and various data sources.
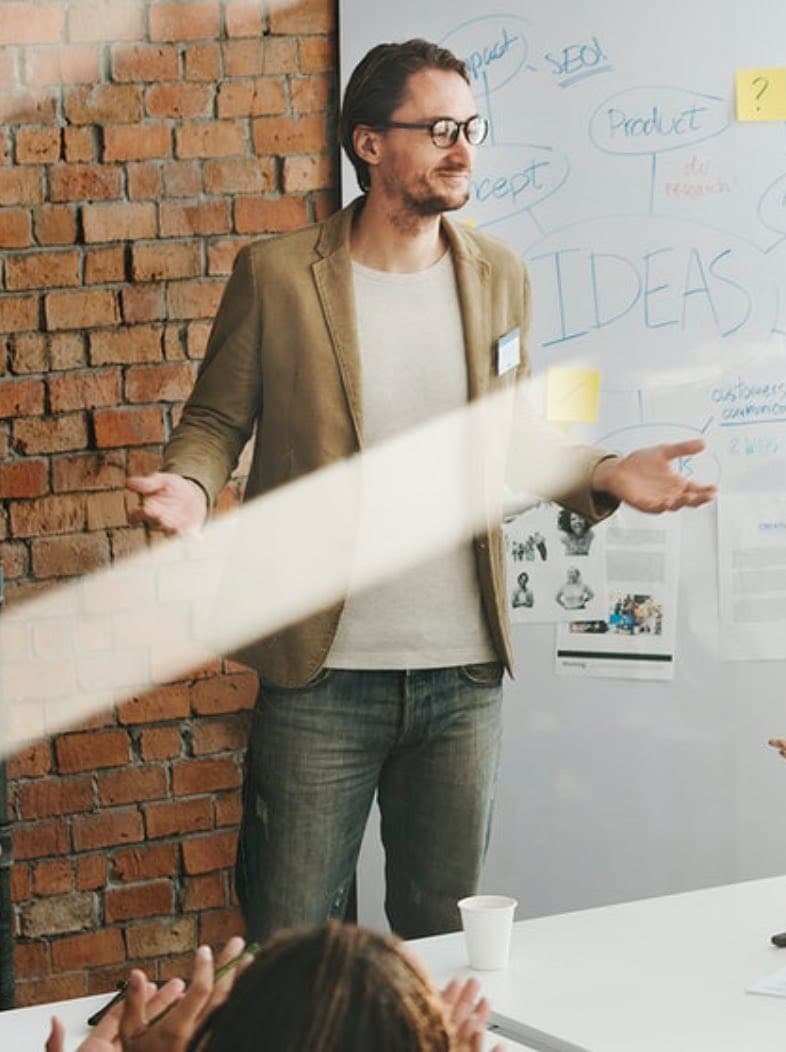
Data Quality & Integrity
Users lose confidence and trust in data
Poor data quality can lead to incorrect decisions and wasted resources Ensuring data is accurate, complete, and consistent builds trust.
Merging data from different sources and systems can be a complex and time-consuming task; but required for a complete picture to make informed decisions.
Ensuring that data is being used and stored securely is required to protect sensitive information and meeting regulatory requirements.
Infrastructure & Automation
Large technical debt burden continues to grow

Messy, complex, and legacy data storage environments
As the amounts of data to manage rapidly increase, companies increasingly face problems when trying to organise and integrate their data in a single source-of-truth environment that is easy to manage and scale.

Poorly scalable legacy data management platforms
As technical debt continues to grow with each day, current systems continue to be expensive to maintain & operate.

Lack of data security, resiliency, and infrastructure automation
Enterprises must eliminate manual intervention to create an error prone system and implement holistic event monitoring or alerting
Monetization
The cost center reputation is poised for transformation

Data systems continue to be cost centers, rather than revenue generating
- Offer standardize reports or anonymized research data sets that enable customers to up-sell or cross-sell.

Reports are reactive and reflective of the past
- Transact on real-time artificial intelligence processing that yields data-driven actions & insights.
Data Modernization Services

Data Assessment & Planning
Assessing and aligning business objectives and priorities to a modern data management strategy – including infrastructure, tools & technology, and daily enterprise operations.

Data Architecture
By aggregating, integrating, structuring, and storing data from disparate sources to provide a consolidated view of your business data, an architecture is designed to deliver on strategic priorities.

Data Engineering
A modern cloud-native architecture with automated ETL/ELT data pipelines that generate high-quality, trustworthy data through data cleansing, profiling, normalization, extraction, and transformation.

Data Modeling
Enabling customers’ data scientists with relational models for reporting and analysis purposes – including training AI/ML models regardless of volume, veracity, velocity, and variety of the data itself.

Data Migration & Integration
Migrate, transform, and optimize legacy data stores to a modern and secure cloud-native architecture to unlock value from its data. Supporting real-time, near real-time, and batch integrations with necessary systems.

Continuous Integration & Development (CI/CD)
Ensure data management environment is DevOps-enabled for proper organization, up-to-date, consistent as changes are incorporated on an on-going basis, and error-free data flow.